Predicting Landslides
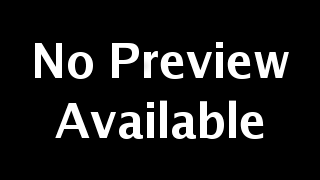
When the rain falls, the land slides.
If a slope's underlying foundation is unstable, heavy rainfall could be all it takes to trigger a landslide. In fact, rainfall is the most common catalyst for landslides. An open sourced computer model developed at NASA's Goddard Space Flight Center uses precipitation data to identify areas all over the globe that are potential landslide hazards. The model first looks at areas that have recently experienced heavy rainfall using data from the Global Precipitation Measurement (GPM) mission. When the rainfall estimates are unusually high, the model checks other known conditions of the area that may encourage landslides, such as recent road construction, steep hills, and other factors. A "nowcast" map then combines the rainfall data and these other factors to mark areas that might have landslides. Scientists have used these models to conduct studies on long term landslide patterns and landslide warning signs. Watch the video to learn more.
Learn how the Landslide Hazard Assessment model for Situational Awareness tracks potential landslide activity over time.
Each month's potential landslides are shown in blue to red, with purple dots indicating reported landslide fatalities.
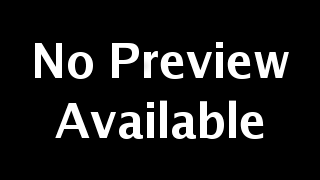
The purple dots show the distribution of fatalities between 2007 and 2018 that are related to rain-fall triggered landslides.
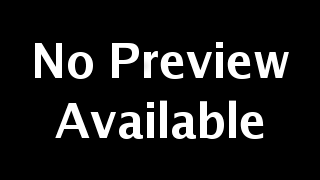
White dots show where a landslide occurred between 2007 and 2018 but with zero fatalities.
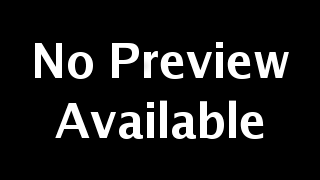
Southeast Asia’s potential landslide activity in any given July shows high risk areas (red) associated with reported fatalities.
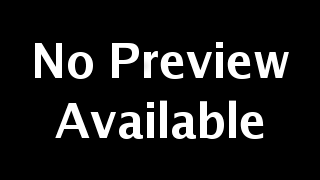
Landslides on N. America’s West Coast in July 2018 are associated with low fatalities and consistent with a history of non-fatal landslides.
For More Information
See NASA.gov
Credits
Please give credit for this item to:
NASA's Scientific Visualization Studio
-
Animator
- Helen-Nicole Kostis (USRA)
-
Scientists
- Dalia B Kirschbaum (NASA/GSFC)
- Thomas A. Stanley (USRA)
-
Producers
- Joy Ng (USRA)
- Ryan Fitzgibbons (USRA)
-
Writers
- Kasha Patel (NASA/GSFC)
- Michelle Tu (Experiential Learning Program, UMD)
Release date
This page was originally published on Monday, July 1, 2019.
This page was last updated on Wednesday, May 3, 2023 at 1:45 PM EDT.